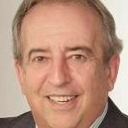
2 weeks ago
SteveDenham
Jade | Level 19
Member since
06-23-2011
- 3,973 Posts
- 2,213 Likes Given
- 254 Solutions
- 2,418 Likes Received
About
Linear models
-
Latest posts by SteveDenham
Subject Views Posted 556 2 weeks ago 142 2 weeks ago 639 2 weeks ago 602 2 weeks ago 607 2 weeks ago 688 2 weeks ago 401 3 weeks ago 687 3 weeks ago 500 4 weeks ago 1080 a month ago -
Activity Feed for SteveDenham
- Got a Like for Re: Repeated measures model executes in MIXED but not in GLIMMIX. a week ago
- Liked Re: Help with Linear Regression for Season. 2 weeks ago
- Posted Re: Repeated measures model executes in MIXED but not in GLIMMIX on Statistical Procedures. 2 weeks ago
- Posted Re: Model heteroscedasticity directly or use log transformation on Statistical Procedures. 2 weeks ago
- Got a Like for Re: Repeated measures model executes in MIXED but not in GLIMMIX. 2 weeks ago
- Liked Re: Help with Demographics Table for FreelanceReinh. 2 weeks ago
- Posted Re: Model heteroscedasticity directly or use log transformation on Statistical Procedures. 2 weeks ago
- Posted Re: Repeated measures model executes in MIXED but not in GLIMMIX on Statistical Procedures. 2 weeks ago
- Posted Re: Repeated measures model executes in MIXED but not in GLIMMIX on Statistical Procedures. 2 weeks ago
- Got a Like for Re: Repeated measures model executes in MIXED but not in GLIMMIX. 2 weeks ago
- Got a Like for Re: Repeated measures model executes in MIXED but not in GLIMMIX. 2 weeks ago
- Posted Re: Repeated measures model executes in MIXED but not in GLIMMIX on Statistical Procedures. 2 weeks ago
- Liked Re: Why ESTIMATION with solely observed data in PROC MI using monotone regression is different for SAS_Rob. 2 weeks ago
- Liked Re: Regression analysis when model assumptions are not met for PaigeMiller. 2 weeks ago
- Liked Re: Regression analysis when model assumptions are not met for PaigeMiller. 2 weeks ago
- Liked Re: WARNING: Ridging has failed to improve the loglikelihood. for StatDave. 2 weeks ago
- Got a Like for Re: WARNING: Ridging has failed to improve the loglikelihood.. 3 weeks ago
- Liked Re: WARNING: Ridging has failed to improve the loglikelihood. for StatDave. 3 weeks ago
- Posted Re: How to do nested counts on SAS Procedures. 3 weeks ago
- Posted Re: WARNING: Ridging has failed to improve the loglikelihood. on Statistical Procedures. 3 weeks ago
-
Posts I Liked
Subject Likes Author Latest Post 1 4 5 1 1 -
My Liked Posts
Subject Likes Posted 1 2 weeks ago 1 2 weeks ago 2 2 weeks ago 1 3 weeks ago 2 4 weeks ago
02-18-2010
07:22 AM
Shoot. Now we're into analytical chemistry, and that's one of the reasons I changed my major back when mammoths roamed the earth.
I'm going to go out on a very thin limb, and guess that the grouping of samples into discrete populations is a matter of chance, and this whole thing might be solved with a larger experiment. That provides absolutely no help in analyzing the data at hand, though. An investigation into lab procedures is about all I could hope to offer, at this point. Perhaps there is some systematic difference in sample prep that leads to the separation.
Maybe someone who has more experience in gage methods will drop by and have something good to offer.
SteveDenham
... View more
02-17-2010
08:15 AM
I don't think the latter idea is going to give you what you need. The fixed effect of sample is probably the best thing that could happen. This should eliminate the bimodal distribution in the residuals, which is problematical. We use mixed models all the time on samples that are bimodal--just consider body weights in a mixed gender population. The males have a different mode/mean than the females, while the distribution around the means is about the same. This is not a problem, if we include gender as a fixed effect in the model. The estimate of the gender effect (males - females) is the difference between the modes/means.
Plus, it confirms (somewhat) my suspicions from the beginning--that there was an unidentified factor separating the measurements into two populations.
SteveDenham
... View more
02-17-2010
08:00 AM
Check out Chapter 12 in SAS for Mixed Models, 2nd ed. Also a google search on Stroup "mixed models" power will be helpful.
SteveDenham
... View more
02-12-2010
08:23 AM
I'll add on to Peter's comments. I think there is a another variable that may not be obvious (and possibly not in the dataset) that separates the two modes. I doubt it is as simple as sex, but failure to recognize some factor like that will lead directly to the situation you have encountered--bimodal variables with bimodal residuals. I like Peter's suggestion of subpopulation analyses as a way to attack this, at least for a first pass.
SteveDenham
... View more
01-12-2010
08:00 AM
Thanks, Dale. That tip should save us a considerable amount of time and effort!
... View more
01-11-2010
08:06 AM
Either
random person(class school)
or
random person(class*school)
will work.
... View more
11-20-2009
11:23 AM
You may need to check your log. When I ran the copied code, I got a missing value for grade, but all the predicted probabilities were present.
Steve Denham
Associate Director, Biostatistics
MPI Research, Inc.
... View more
10-22-2009
07:45 AM
I love "A-ha" moments. I prepared the following, and thought it a neat approach, but then the "A-ha" occurred shortly before posting. I offer the following in quotes, and give the "A-ha" afterwards.
"I'm curious as to why you can't find this. If X is a random value from the univariate distribution on [0,1] and Y is 1-X, then I would think that the formula (via a Taylor's series expansion) could be applied:
E(X/Y) = E(X)/E(Y) * [1 + (V(Y)/(E(Y)^2) - (cov(X,Y)/E(X)*E(Y))]
where E(a) is the expected value of a, V(a) is the variance of a, and cov(a,b) is the covariance between a and b. By using the sample values for each run of the simulation, approximate values of E(X/Y) could be calculated, and then averaged across all runs. It seems like a perfect bootstrap opportunity. I must be missing something here."
Well, I missed a key something. The OP wants the short divided by the long. Consequently, X and Y as I tried to define them do not meet this definition. Sometimes X>Y, sometimes Y>X, so they do NOT define the short and the long pieces. I suppose that if you think about this hard enough, you see that the ratio would follow some kind of Cauchy distribution, implying that the expectation doesn't exist. One could simulate as much as you want, but the thing you end up calculating? I don't know what to call it.
Cool.
Steve Denham
... View more
09-15-2009
12:50 PM
Well, I mangled the first answer and hopefully it was swallowed up whole.
This all comes down to the question you are trying to answer. If you really are interested in comparing levels of factor A at each level of factor B, you have to include the interaction A*B in the model, whether or not it is "significant."
If you drop the interaction, the solution that leads to the LSMeans is the same at each level of factor B for factor A, and similarly for each level of factor A for factor B. That's what your model says is happening--there is no difference by level for the second factor. One size fits all.
Clear as mud, huh?
Steve Denham
... View more
09-11-2009
08:23 AM
I'll try for both answers at once. First, the order is critical for the Kronecker product. The first term is always unstructured, while the second may be unstructured (UN), autoregressive (AR(1)), or compound symmetric (CS). Think about which is most appropriate. Your choices for structures seem logical to me.
The second point--the inclusion of the repeated subject in the random statement--for covariate structures with a correlation term (see the TYPE= tab in the documentation) comes from the paper "Statistical Analysis of Repeated Measures Data Using SAS Procedures" Littell et al. J. Anim. Sci. 1998. 76:1216–1231, and has to do with getting proper standard errors when using structures like AR(1) that involve estimating a correlation. Nothing about a random sample, really.
Message was edited by: SteveDenham
... View more
09-10-2009
07:59 AM
SAS-L archives.
probably the fastest is to go to Google groups, and search the group
comp.soft-sys.sas
the listserve is hosted by the university of georgia, so a google search on those terms should lead to the actual archives.
good luck,
Steve Denham
... View more
09-10-2009
07:54 AM
Wow. This is a very information dense post, and I don't think I can address all of it, so let's try some pieces.
First, nesting in SAS. You need to look at the documentation for MIXED or GLM that covers this. The parameterization of A nested in B and A crossed with B is the same. That is why it makes no difference in the rcorr. Here is what the online manual says:
Nested Effects
Nested effects are generated in the same manner as crossed effects. Hence, the design columns generated by the following two statements are the same (but the ordering of the columns is different):
model Y=A B(A);
model Y=A A*B;
The nesting operator in PROC MIXED is more a notational convenience than an operation distinct from crossing. Nested effects are typically characterized by the property that the nested variables never appear as main effects. The order of the variables within nesting parentheses is made to correspond to the order of these variables in the CLASS statement. The order of the columns is such that variables outside the parentheses index faster than those inside the parentheses, and the rightmost nested variables index faster than the leftmost variables (Table 56.18).
Table 56.18 Example of Nested Effects Data
(I deleted the table as it doesn't come across very well)
Note that nested effects are often distinguished from interaction effects by the implied randomization structure of the design. That is, they usually indicate random effects within a fixed-effects framework. The fact that random effects can be modeled directly in the RANDOM statement might make the specification of nested effects in the MODEL statement unnecessary.
The other part that I want to address is the doubly repeated measures. This assumes that the correlation of residuals amongst days is the same, no matter which period we have. I was thinking that observations on the close time points would be more highly related, and probably similar, so that an ar(1) or cs structure might fit. The separated time points (i.e., periods) would have a different relationship.
If you want to deal with all at once without those assumptions, you have unequal spacing and the spatial power method goes after that with the assumption that the errors are "constantly correlated" as in ar(1), but that the points used to estimate that correlation are not equally spaced.
A read of the TYPE= section of the PROC MIXED documentation might point you at the right kind of structure.
Good luck on all of this.
Steve Denham
... View more
09-02-2009
08:07 AM
Aha. Now I understand part of what is going on. Think about the repeated statement:
repeated day(period)/subject=animal(farm) type=cs;
and how SAS will parameterize this statement. The parameterization is the same as:
repeated day*period/subject=animal*farm type=cs;
This makes sense to me that you would get the same results if you respecify as you mention. Try adding the rcorr option after the slash, and take a look at the correlations under your various approaches. They should be identical, as they are restatements of the same approach.
Some alternate approaches might be:
repeated day*period/subject=animal*farm type=csh
repeated day*period/subject=animal*farm type=sp(pow)(time)
where time is actual elapsed days post study start
or even:
repeated period day/subject=animal*farm type=UN@AR(1)
which would model an unstructured relationship between periods, but a common autoregressive relationship within periods.
Note also that for the latter two structures, you should include animal*farm in the random statement, so that between animal variability is correctly modeled.
Good luck.
Steve Denham
... View more
09-02-2009
07:52 AM
Wow. What a cool problem.
I don't have any answers, but maybe some observations that might help. It looks like a doubly repeated measures design. You might try a Kronecker product as the covariance structure--something like UN@AR(1). Unfortunately, this isn't available in GLIMMIX. If you are looking at SAS for Mixed Models, there is a section on unequally spaced timepoints (3.5 in the first edition, 5.4 in the second), where they talk about using spatial correlations, so maybe sp(pow)(time) would work. This structure is available in GLIMMIX.
As far as distributions, before you start looking at zero inflated processes, first check a negative binomial--it may be that the data is just overdispersed for a poisson distribution. However, if you are going into ZI processes, search the SAS-L listserve archives for this topic, especially articles by Dale McLaren (stringplayer_2@YAHOO.COM>). It will lead you to NLMIXED as a possibility for this sort of data.
Finally, if that seems like overkill, consider MIXED and an appropriate transformation. For counts, I seem to remember that a square root transform is variance stabilizing, or you might try log(counts+1).
Good luck.
Steve Denham
... View more
08-28-2009
08:22 AM
1 Like
GLM always treats variables as continuous and as coming from a normal distribution. It doesn't use a Z test. The Z test assumes that you have a known variance, whereas a t test, and linear models in general, uses the sample variance as an estimator. In answer to your question, "how does it handle categorical/binomial dependent variables", the short answer is: It ignores the fact that the variable is categorical or binomial. All responses are treated as continuous. For binomial responses, we have seen that this isn't too bad in a lot of cases, because we can sort of rank a yes/no response. For true categorical variables, such as product brands, or various politicians, this can't really be done, and GLM is likely to give bad results.
SAS has other procedures that are more appropriate for these sorts of distributions--LOGISTIC, GENMOD, GLIMMIX--that use the tools of linear models and recognize the distribution of the outcome variables. But these are "newer", and utilize methods that usually are not covered in intro stat courses. Consequently, GLM or TTEST are the tools that people have seen. And to quote a famous proverb, "When the only tool you have is a hammer, every problem looks like a nail." People go around hammering--sometimes the results aren't so bad, sometimes you break the crockery.
... View more
- « Previous
- Next »